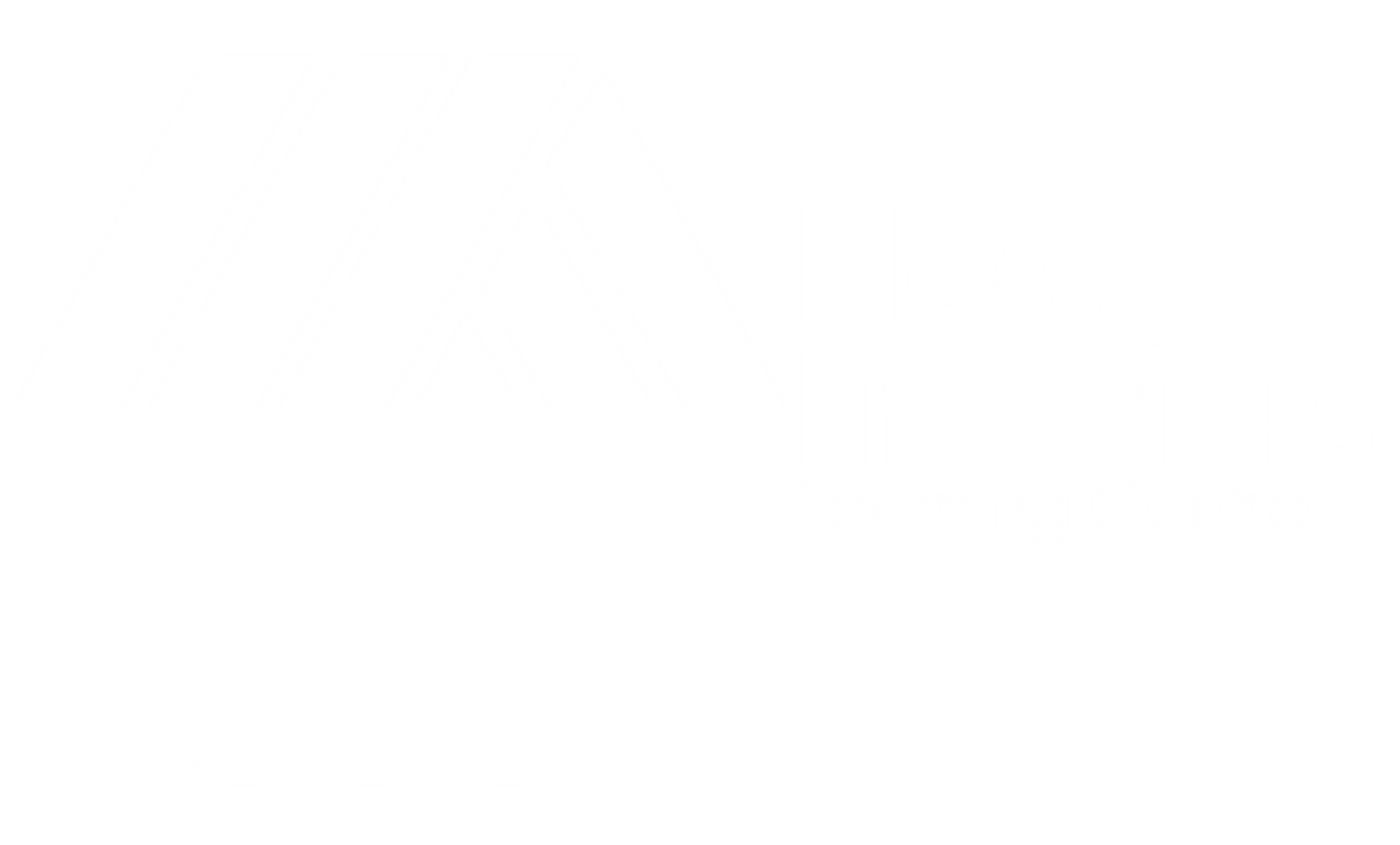
This study session provides coverage on how linear regression and time-series analysis are used as tools in financial analysis for identifying relationships among variables. The session begins by examining linear regression with a single (independent) variable to explain or predict the value of another (dependent) variable. Multiple regression, using more than one independent variable to explain or predict a dependent variable, is explored next. Time-series analysis, in which the dependent variable’s past values are included as independent variables, concludes the session.
This study session provides coverage on techniques that underlie how financial technology (fintech) is affecting areas within the investment industry, such as investment analysis, automated advice, and risk management. The first reading introduces techniques in machine learning (ML) that involve clustering, simplifying, classifying, and predicting relationships in the large datasets that are often found in finance. The next reading examines how data projects involving large datasets are structured with an application to sentiment analysis in investment analysis using machine learning techniques for natural language processing (NLP). The session concludes with coverage of probability-based techniques for assessing risk, with a focus on simulation models.
20B Amodu Tijani Close Off Sanusi Fafunwa,
Victoria Island, Lagos.
+ 234 817 0634 754
newfrontierslearning@gmail.com
Copyright © 2020 New Frontiers Learning, All Rights Reserved.